LivePerson, Inc. (NASDAQ:LPSN) Q4 2022 Earnings Call Transcript March 17, 2023
Operator: Good afternoon, ladies and gentlemen. Thank you for standing by. Welcome to LivePerson’s Fourth Quarter 2022 Earnings Webcast. As a reminder, this conference is being recorded. I would now like to turn the conference over to Mr. Chad Cooper, Senior Vice President, Investor Relations.
Chad Cooper: Thank you, operator. Joining me on the call today is Rob LoCascio, LivePerson’s Founder and CEO; John Collins, Chief Financial Officer; Joe Bradley, Chief Data Scientist; and Dr. Matt Dawson, Founder and CEO of WildHealth. Please note that during today’s call, we will make forward-looking statements which are predictions, projections and other statements about future results. These statements are based on our current expectations and assumptions as of today, March 15, 2023 and are subject to risks and uncertainties. Actual results may differ materially due to various factors, including those described in today’s earnings press release and in the comments made during this conference call as well as in 10-Ks, 10-Qs and other reports we file from time to time with the SEC.
We assume no obligation to update any forward-looking statements. Also during this call, we will discuss certain non-GAAP financial measures. A reconciliation of GAAP to non-GAAP financial measures is included in today’s press release. Both the press release and the supplemental slides, which include highlights for the quarter, are available on the Investor Relations section of LivePerson’s website. With that, I will turn the call over to Rob. Rob?
Robert LoCascio: Thanks, Chad, and thanks, everyone, for joining us for the Q4 2022 quarterly call. We’ll be here with John Collins, our CFO; and Joe Bradley, also, our Chief Data Scientist, will be joining us to talk about our AI platforms. And then we also have Dr. Matt Dawson, who is the Founder and CEO of WildHealth, and he’ll be talking about everything we’re doing on the AI health care initiatives. Now there’s been a lot of excitement around AI, especially with everything that’s going on with ChatGPT since November. And obviously, the consensus is that this is not an evolution of technology, but a revolution. And for LivePerson, this is a profound time in our history as we have waited over 20 years for this day to come and we have spent the last 5 years investing heavily in being a leader in AI in the enterprise AI space.
We’ve had a vision for a very long time that humans, that we could talk to a machine and that machine could help us, through natural language, solve our most important aspects of our life, things in our health and things around our finances and our relationships with our brands that sometimes get strained when we’re sitting on calls on hold. And so we made a big bet 5 years ago, and now we’re here as one of the few companies in the world that can go after this opportunity as an AI company, especially in the enterprise. Now we spent the last year getting the house in order and creating a foundation to go after this opportunity. And we’ve created now a model for the business that I think will allow us to go back to where we came from in generating EBITDA, generating cash flow.
And I’m confident that the trajectory of what’s about to happen to us is going to be built on a solid foundation. And obviously, over the COVID time, there was a lot of things that happened to our company and others where we invested into areas. We went after different opportunities with our customers, but Q4 and Q1 is a place where there was a lot of noise, but we’ve all addressed and resolved those noises. And now what we’re going to do is, starting in Q2, we have a new company. We have a company that will generate, I think, strong earnings, cash flow, EBITDA, and from there, we’ll go after this massive opportunity. Now as we mentioned in previous calls, we’re really focused on getting rid of non-core revenues. These are revenues that don’t recur.
These are revenues that are not supporting the core vision that we have around AI, and Q1 will be our trough in revenue, in EBITDA and cash flow. And then from there in Q2, there will be an inflection point up, EBITDA will be positive, cash flow will be positive, and we will continue growing top line and bottom line throughout the year. The company as well as our shareholders will benefit, I think, from simplifying the business and being more transparent about our revenues. And already and starting Q2, we’ll do a couple of things, and John will go into them in a minute, but we’re going to focus on the recurring revenue lines. We have about $340 million of recurring revenue. That’s 80% of our total revenue. Now the growth rates in that are single to mid-single digits.
But obviously, we’ve got the wind in our sales to bring that to another level. Obviously, we balanced out how do we get to a strong business model, generate cash with growth. But from there, we can grow now at a different level. We’re also focused on achieving annualized EBITDA margins of 16% to 19% by year-end. We’ll start generating positive margins, as I said, in Q2, and we’ll also start generating positive cash flow in Q2, targeting 7% to 10% cash flow by year-end. Those are facts. Those are things that will carry forward. Those are things that we had in this company for over 20 years, and that’s what got us here, and that’s what will get us to where we need to go now with this exciting opportunity in our hands. What I’d like to do now is hand it over to John Collins, who can do a deeper dive on the P&L and really show you what’s making up the base that’s allowing us to go after this big opportunity.
John?
John Collins: Thanks, Rob. During the past 12 months, we have rationalized our cost structure, yielding an expected 36% or $200 million in reduction of annualized costs. We also wound down non-core business lines in order to enable profitable growth and sharpen our focus on LivePerson’s B2B core software business, including the AI technology that powers it. As I’ll elaborate on shortly, we have a strong base of recurring software revenue from top brands across the globe that is expected to grow, as Rob said, in the low to mid-single digits and represent approximately 80% of total revenue in 2023. Our success in reducing costs last year, coupled with additional cost reductions in the current quarter, is expected to yield double-digit adjusted EBITDA margins and positive free cash flow for the B2B core beginning in the second quarter.
On an annualized basis, we expect the B2B core to exit the year with at least a 16% adjusted EBITDA margin and up to a 10% free cash flow margin despite the expected decline of more than $70 million in non-core revenue on a year-over-year basis, most of which was set in motion by the profitable growth strategy that we launched last year. In short, we’ve materially improved the P&L and reallocated resources to focus on the B2B core, establishing a solid foundation to execute on AI-led growth in the second quarter and beyond. With this overview of the B2B core P&L in mine, we think investors and analysts would benefit from additional transparency into our total company revenue stack, particularly the non-core components of the P&L that we began winding down last year.
Beginning with total company revenue in 2022, of the $514.8 million that we recognized, again, over $70 million was non-core revenue that we do not expect to reoccur in 2023. Breaking that down, of over $50 million of the non-core revenue year-over-year revenue decrease, falls into 2 related categories: COVID-19 testing; and to a larger extent, professional services with a strategic partner in the diagnostics space. We now expect professional services, including a large software build, to complete ahead of schedule in the first quarter. Setting aside approximately $3.8 million of professional services revenue tied to the conclusion of the diagnostics project in the first quarter, we expect professional services revenue in 2023 to be exclusive to the B2B core and consistent with its historical relationship to expansions and new logos.
Next, over $20 million of the non-core year-over-year revenue decrease relates to Gainshare labor and pandemic-driven variable revenue, which we discussed as not strategic to the B2B core on the second quarter call last year. The largest remaining source of revenue in this category is expected to exit the P&L in the second quarter of 2023. As discussed on the prior two quarterly calls, the nonlabor software component of our Gainshare business has almost fully converted from variable to recurring revenue and represents committed usage of the conversational cloud that is identical to software consumption by our other B2B core customers. For this reason, e classified this software revenue as B2B core revenue, representing approximately 5% of total recurring software revenue, and we will no longer refer to Gainshare customers in the context of the B2B core.
Finally, and consistent with the sharpened focus on the B2B core, we have classified the business underlying the consumer segment as held for sale in our financial statements, with a targeted transaction close date before the end of this month. To summarize the key points directly, we have materially improved the P&L and we are drawing a line at the first quarter, after which we expect the B2B core’s performance to be transparent and its business drivers consistent with growing revenue by increasing the Conversational Cloud’s share of enterprise to consumer engagement, especially AI-based engagement across both voice and master. Turning to the fourth quarter of 2022. There were 2 unanticipated changes that impacted our results. First, as disclosed last week, we received notice in November that Medicare reimbursement for a non-core WildHealth program was suspended pending further governmental review.
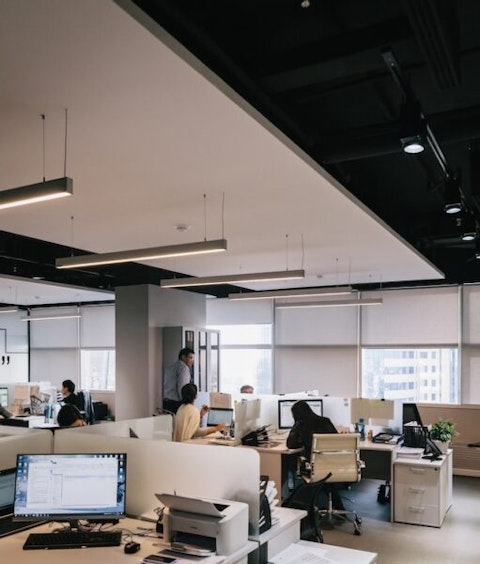
Photo by Damir Kopezhanov on Unsplash
We currently believe that the services rendered under the program in the fourth quarter of 2022 were valid and should ultimately be reimbursable. However, in view of the inherent uncertainty as to the timing and amount of further reimbursement, we have elected to take the most conservative position by not recognizing revenue for which reimbursement has not yet been collected. I’ll also note that the services under this program were subsequently discontinued, consistent with the focus on exiting non-core activities. But for this event, revenue would have been within our guidance range for both the fourth quarter and the full year of 2022. Second, in light of the remaining capacity under our 2019 stock incentive plan and in order to mitigate dilution to our shareholders, especially in light of current valuations and, more generally, to improve the quality of earnings, we’ve decided to pay the 2022 employee bonus in cash instead of stock.
Note that in recent years, we have paid annual employee bonuses in stock, which was the original plan for 2022. But for the WildHealth revenue reserve and this decision to change the method of settlement for the 2022 employee bonus, adjusted EBITDA would have also been within our guidance range for both the fourth quarter and the full year of 2022. With the impact of these changes, revenue for the fourth quarter and the full year was $122.5 million and $514.8 million, respectively. Adjusted EBITDA, reflecting these impacts for the fourth quarter and full year, was a loss of $5.2 million and $16.2 million, respectively. With that, I’ll turn to our reporting segments. For the fourth quarter, within total revenue, B2B revenue declined approximately 1% year-over-year, while revenues from our hosted software declined 8% year-over-year.
Excluding the impact of COVID-19 testing and pandemic-driven Gainshare variable revenue, B2B grew 6% year-over-year and hosted declined 1% year-over-year. Professional services revenue grew 30%, driven primarily by the diagnostics project that I referenced earlier. The consumer segment declined 3% due primarily to decreased marketing spend in the quarter. From a geographic perspective, U.S. revenue grew 3% year-over-year, and headwinds in EMEA caused international revenue to decline 8%. We signed a total of 90 deals in the quarter, including 1 7-figure deal, 46 expansions and renewals, 44 new logo deals. While the total number of deals decreased year-over-year, the enterprise deal count increased 22%, and the new logo deal count increased 63%.
In general, however, we observed more deals but at lower-than-expected dollar values, indicating that some macroeconomic impact may have compressed budgets in the fourth quarter. To highlight a few of these deals, we re-signed a top 3 global airline to a 2-year contract, which is using us for a wide array of service use cases, including refunds, baggage, reservations and travel credits. We also re-signed one of the top online travel companies to a 2-year extension and re-signed a top 25 financial holding company in the U.S. to a 2-year extension. For new logos, our APAC team had a strong quarter with the signing of an Australian multinational banking and financial services company as well as one of the leading providers of pension and investment products in Australia.
As for net revenue retention, consistent with our focus on the B2B core and recurring revenue growth in particular, and in light of the more than $70 million in non-core revenue that we do not expect to reoccur in 2023, we think investors and analysts would benefit more from net retention for recurring revenue than from net retention for total revenue. For this reason, we are providing both net retention metrics for the fourth quarter and expect to provide only net retention for recurring revenue in the first quarter of 2023 and beyond. In the fourth quarter, net retention for total revenue was well below our target range of 105% to 115%, driven as expected by lower Gainshare variable and labor revenue and the elimination of COVID-19 testing revenue.
Net retention for recurring revenue was above 100%, but below our target range of 105% to 115%. Finally, average revenue per customer was $680,000 in the fourth quarter, up 11% year-over-year. As I discuss guidance, note that there was a clerical error in our press release that was just issued with respect to guidance. The guidance I’m about to provide is correct in the press release. An 8-K will be reissued as soon as possible to reflect these corrections. For total company metrics in the first quarter of 2023, we expect total revenue to range from $106 million to $109 million or a decrease of 19% to 16% year-over-year. For adjusted EBITDA, we expect a loss in the range of $8 million to $6 million. The year-over-year decrease in revenue and the sequential decrease in adjusted EBITDA are both driven primarily by the large amount of non-core revenue that is exiting the P&L beginning in the first quarter.
The largest drivers in the first quarter are decreasing Gainshare labor and variable revenue and decreasing professional services from the strategic partner in the diagnostics space that I referenced earlier. As for total company metrics for full year guidance, we expect total revenue to range from $422 million to $436 million or a decrease of 18% to 15% year-over-year; and adjusted EBITDA to range from $15 million to $32 million or a margin of 4% to 7%. Consistent with the themes of transparency and focus on the B2B core, we also think providing guidance for our B2B core recurring revenue and adjusted EBITDA would be instructive for investors and analysts. For the first quarter of 2023, we expect recurring revenue to range from $80 million to $83 million or a decrease of 7% to 3% year-over-year.
For adjusted EBITDA, we expect a loss in the range of $6 million to $4 million. As previously discussed, in view of the cost reductions that will be effective this month, we expect the B2B core to produce double-digit adjusted EBITDA margins and positive free cash flow beginning in the second quarter and to improve sequentially throughout the year. For the full year, we expect recurring revenue to range from $334 million to $347 million or 0% to 4% growth year-over-year; and adjusted EBITDA to range from $27 million to $40 million or a margin of 7% to 11%. On an annualized basis, we expect the B2B core to exit the year with a 16% to 19% adjusted EBITDA margin and a 7% to 10% free cash flow margin. Before passing the call back to Rob, I’ll emphasize a few key points to conclude.
Relative to the first quarter of 2022, when we launched our plan to achieve a balanced approach to profitability and growth, we’ve reduced our cost structure by an expected 36% or $200 million in annualized costs. By winding down nonstrategic and low-margin lines of business, we have also provided immediate clarity on LivePerson’s foundation, the B2B core business, driven by a growing base of software recurring revenue that represents approximately 80% of total company revenue in 2023. Beginning with the second quarter, we expect B2B core to be profitable, generating double-digit adjusted EBITDA margin and a positive free cash flow. We also expect AI-led growth in recurring software revenue to further improve profitability throughout the year to an annualized rate of at least 16% in terms of adjusted EBITDA and up to 10% in terms of free cash flow, further strengthening both our balance sheet and the P&L.
And with that, Rob, I’ll hand it back to you.
Robert LoCascio: Thanks, John. Obviously, we took the opportunity. There’s a lot of noise and a lot of puts and takes, but we put the past in a box, and now we have a foundation come Q2. And what I want to do is talk about what’s next and what do we build from that foundation based on our strategy around AI that we’ve been pursuing for over five years. What I want to do is first talk about what our strategy is and address it from really three perspectives. One is a strategic perspective, an engineering product perspective and an industry perspective around our view of AI and how we’re going about it. From a strategic perspective, AI has two foundational pieces. One is the data models. And that’s the stuff we’re seeing with OpenAI and large language models.
And the other, just as important, is actually the data set that the model uses to generate outcomes. There’s been a lot of focus on the model because of what’s gone on with ChatGPT. But we can see sometimes it has issues because the underlying data set is using public data. LivePerson has one of the largest and most precise conversational business data sets in the world. And I use the word precision because over 350,000 people on our platform each day, and they’re messaging their live agents and they are generating 1 billion conversations a year, and these are high-quality conversations around many different topics, but the conversations are precise. There are answers to questions in the way the brand would want it answered. These agents, they create a long tail of answers.
Today, as we said in the past, we automate fully about 20% to 30% of the conversations that are on our platform. About 75% of conversations have some form of AI, but end-to-end automation is about 20% to 30%. With what’s happened with large language models and what we’re doing with them is that we can go after a much larger pool of intents. We can go after the long tail of intents that’s sitting in our data today, and we believe we can automate 80% to 90% of conversations, not only on messaging, but in voice. We are bringing our voice AI product. It’s weeks away now, and we’re basically going to be able to go after both channels. So we sit in a very unique space to power the enterprise at scale and in a safe way with large language models, and we are going to accelerate what we’re doing.
Now from an engineering perspective, we began developing our own AI capabilities 5 years ago. And today, 50% of our engineering budget is on AI and automation. And this is backed by world-class team of product and engineering leads, data scientists and many who came from the acquisitions we did over the past several years, from BotCentral, from VoiceBase, Tenfold, , WildHealth. As a matter of fact, we recently took one of the founders of BotCentral, who we acquired 5 years ago, and now he’s leading a group that is working with our largest top 100 enterprise customers so that they will adopt this technology at a faster rate. Because what we’re hearing from our customer base is they want to talk. They want to talk to engineers, they want to talk to the product heads because they’re going to buy AI from those people.
And even our sales team, although they’re out there and they’re looking for more new opportunities, our base of customers want the implementation, want the knowledge from the people who built these platforms. So I think the impact of that on our net retention rates and also our ability to grow the company at a different level will be profoundly changed with a restructuring of that business. And then lastly is, from an industry perspective, many of you may know, about four years ago, I founded a nonprofit called EqualAI with Miriam Vogel. Miriam was the Associate Deputy Attorney General under President Obama, and she led the team that developed the implicit bias training programs for federal law enforcement. She recently also has been appointed as a chairperson to President Biden’s National AI Advisory Committee.
And her and I built this nonprofit in this organization. We have a great Board of Directors and it’s focused on one thing: How do you bring AI safely to the enterprise? And there’s a framework and training that Miriam has developed with her team, and LivePerson has been a great supporter of that and we’ve obviously adopted many of those frameworks in our own technology. So what this all boils down to is that we’re not trying to catch the future of AI. We’re actually leading that and we’re leading in our area, which is the enterprise AI space. What I want to do is bring Joe Bradley on, who’s been with us for five years. Joe is our Chief Data Scientist, and I want him to do a deep dive on our platform, our data set, what we’re doing and what we’ve been doing with large language models and then some of the things that are happening recently that will also drive more profitability to the company.
Just a little background before I bring Joe on, before he was here, he was Head of Data Science at Amazon Search and Nike, in addition to spending many years as a physicist and research associate at Lawrence Livermore National Laboratory, and he holds a doctorate in physics. So Joe leads our team of world-class scientists. And with that, Joe, let me turn it over to you. I think it’s the first time that a data scientist has been on a quarterly call, but this is an AI-focused thing. So enjoy.
See also 11 Most Promising Car Stocks to Buy and 11 Best Coal Mining Stocks to Buy Today.
Q&A Session
Follow Liveperson Inc (NASDAQ:LPSN)
Follow Liveperson Inc (NASDAQ:LPSN)
Joe Bradley: Thanks, Rob. Very happy to be here. I’ll put up some visuals here real quick for people. The opportunities that the latest generation of generative AI and large language model technology creates for LivePerson are exciting. Essentially, they open up, as Rob mentioned, a large new addressable market for us. I’m here today to explain a little bit about how that works. Essentially, LivePerson has three core strategic assets for machine-learned model building in general, but which are particularly relevant for this new generation of large language models. First, we have billions of conversations running through the platform. These are what we call goal-oriented dialogues, which just means people solving real problems and they’re full of the real complexities of doing that with human dialogue.
This is one of the world’s richest baseline data sets for an LLM-based conversational system. Second, we already have a rich processing layer for understanding that data, which creates billions of derived data points, including sentiment, conversation quality, user intent, user problem resolution, customer satisfaction, et cetera, much of this using prior and current generations of large language model technology already. It’s part of why we have hundreds of millions of conversations handled today by our own first-party AI products. Third, we have, as Rob mentioned, over 300,000 human experts that log into the platform every day, providing feedback and helping models learn. Each of these assets is uniquely meaningful in this new LLM context.
First, these large language models need baseline data to train their basic behavior. This must be — is typically done with and really must be done with, today, Internet-scale data sets. LP holds one of the few goal-oriented conversational data sets at this kind of scale. Secondly, because each conversation on LP’s data set is already adorned with quality measures, associated business outcomes, et cetera, we can teach these models how to drive towards these outcomes by highlighting particularly successful conversations or by rewarding models differently as the conversation changes. Third, in addition to the scale of the latest generation of large language models, the other key performance driver has become increasingly human feedback through techniques called reinforcement learning.
The largest models in the world are typically trained with tens of thousands or hundreds of thousands of examples of such feedback, and our platform generates this scale of data daily. This is a powerful mechanism for controlling model behavior. It teaches them,- for example, when they might be hallucinating. It teaches them what not to say and when not to say it. This is incredibly important in an industrial context. And this — for all these reasons, this is why we’re partnering with the world’s leading AI organizations in the large language model and generative AI space. In particular, we’re very excited about the global rollout possibilities that we’re seeing in working with Microsoft’s Azure platform. Our assets allow us to move quickly to produce high-precision conversational systems today and to further push the state of the art tomorrow.
More importantly, they allow us to keep these models grounded, factual and aligned with the humans who will use them. What this means for us and for the world, I think, is that LLMs are transitioning from fit-for-purpose models that can understand aspects of the conversation and make specific decisions, into systems that themselves can drive and control a dialogue. We’ve all started to experience that with some of the latest ChatGPT technology, for instance. That’s a big change for us. Up until now, dialogues had to be programmed explicitly in our system and in any thought building system in the world. And this doesn’t scale because dialogues are, by nature, a very long tail process. They’re very unique. But we now have technology that’s capable of handling dialogues in their full complexity and nuance.